The Essential Role of Labelling Tool Machine Learning in Data Annotation
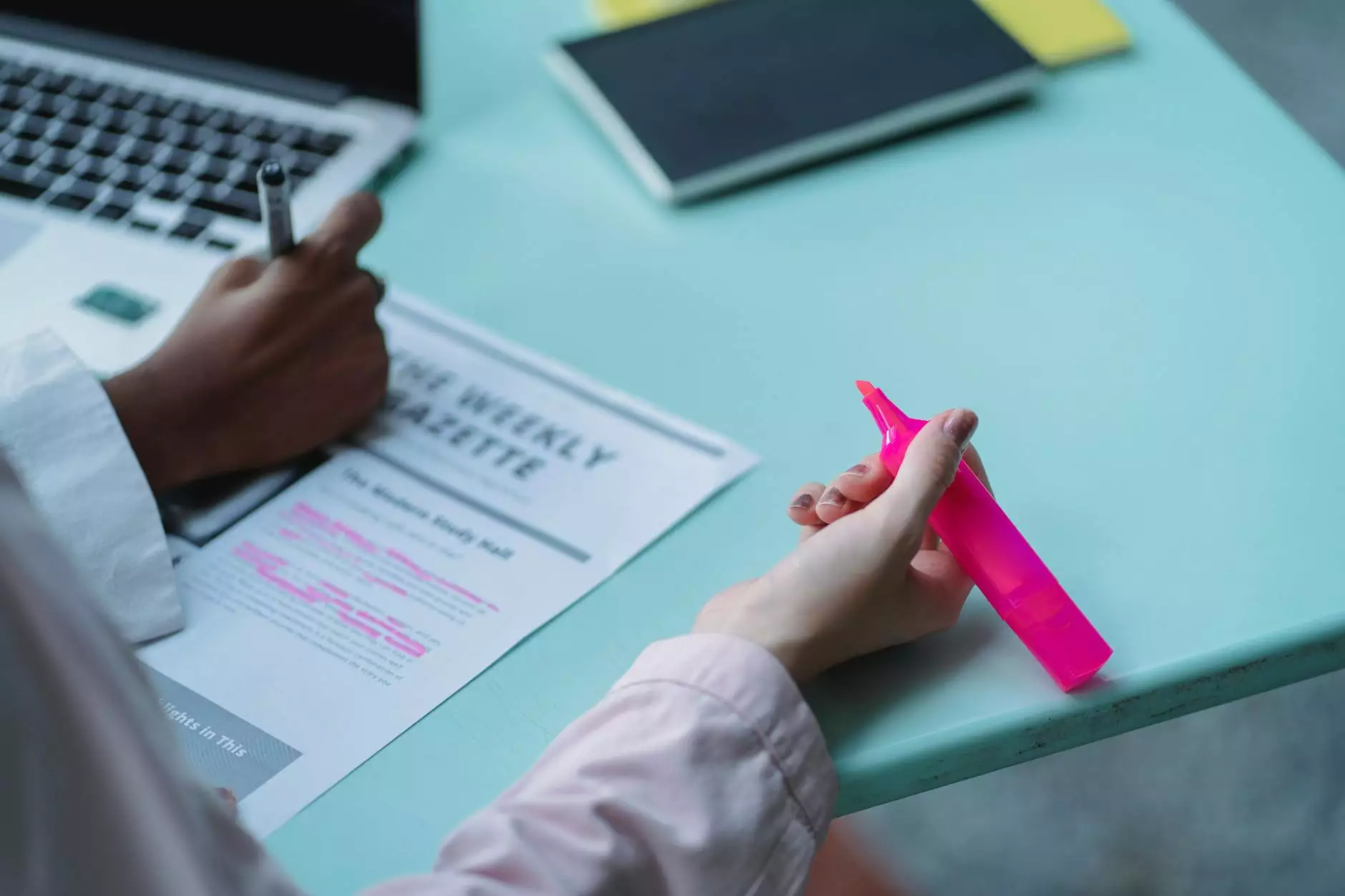
In today's data-driven world, businesses are continually looking for ways to leverage their data for enhanced decision-making. One of the most pivotal components in this journey is effective data annotation. This is where the labelling tool machine learning comes into play, serving as a cornerstone for creating high-quality, annotated datasets that feed machine learning algorithms.
Understanding Data Annotation and Its Importance
Data annotation refers to the process of labeling data so that machine learning models can learn from it. Whether the data consists of images, text, or audio, accurate annotations are crucial for developing reliable and efficient machine learning models. Inaccurate data can lead to flawed predictions, which can severely affect business outcomes.
Businesses across various sectors, including healthcare, finance, automotive, and e-commerce, rely on data annotation to train their algorithms. Annotations provide essential context to raw data, enabling algorithms to recognize patterns, make classifications, and generate insights.
The Rising Demand for Labelling Tool Machine Learning
As companies increasingly harness the power of artificial intelligence, the need for sophisticated labelling tool machine learning solutions is more pressing than ever. Organizations require tools that are not only efficient but also scalable to handle vast amounts of data. Here are some factors fueling the demand:
- Growing Data Volumes: In the digital age, the volume of data generated daily is astronomical. Businesses need powerful annotation tools to keep pace.
- Need for Accuracy: Accurate data annotations are critical to the success of machine learning initiatives. Tools must provide precision and reliability.
- Increased Project Complexity: More complex machine learning projects require enhanced capabilities in data labelling.
- Time Efficiency: Faster data annotation enhances operational workflow, allowing organizations to get their products to market quicker.
Key Features of an Effective Labelling Tool for Machine Learning
When looking for a labelling tool machine learning solution, businesses should consider the following features:
- User-Friendly Interface: A straightforward and intuitive design increases productivity and reduces training time.
- Support for Multiple Data Types: The ability to annotate various data formats (images, text, audio) is essential.
- Collaboration Tools: Features that allow multiple users to work on data simultaneously enhance teamwork and efficiency.
- Integration Capabilities: The ability to easily integrate with existing workflows and systems is crucial for seamless operations.
- Automation Features: Utilizing AI to assist with the labelling process can significantly accelerate workflows.
- Quality Assurance Mechanisms: Tools that include quality control features ensure annotation accuracy.
- Scalability: The tool should grow with the business and accommodate increasing data workloads.
How Labelling Tool Machine Learning Optimizes Data Annotation
Implementing a robust labelling tool machine learning solution can lead to significant advantages in data annotation processes. Let’s explore how these tools optimize operations:
Enhanced Speed and Efficiency
Automation within labelling tools allows repetitive tasks to be handled quickly. For instance, computer vision models can automatically pre-labell images, significantly reducing the time required for human annotators to finish their tasks.
Improved Annotation Quality
With advanced algorithms, machine learning labelling tools can minimize human errors. These tools often incorporate validation processes that ensure each piece of data is accurately labelled and reviewed.
Cost-Effectiveness
By streamlining the annotation process and reducing the time spent on manual tasks, businesses can significantly cut down on operational costs. Investing in high-quality labelling tools also leads to better-performing machine learning models, ultimately improving the bottom line.
Trends Shaping the Future of Labelling Tool Machine Learning
The landscape of data annotation is rapidly evolving. Here are some trends shaping the future of labelling tool machine learning:
- AI-Driven Automation: The future will see an increase in AI assisting in the labelling process, reducing the need for extensive human input.
- Better User Experience: The focus on user-friendly interfaces will continue to grow, enabling faster adoption by teams.
- Focus on Security and Data Privacy: As data privacy regulations become stricter, annotation tools will need to evolve to ensure compliance.
- Integrative Technologies: New partnerships and integrations with other business applications will enhance the efficacy of annotation workflows.
- Customizable Solutions: The demand for tailored solutions that meet specific business needs will increase.
Choosing the Right Labelling Tool Machine Learning Solution
Choosing the right labelling tool machine learning involves assessing your organization’s unique needs. Here are steps to guide you through the selection process:
- Identify Your Requirements: Determine the types of data you need to annotate, required features, and your team size.
- Evaluate AI Features: Look for tools that incorporate advanced machine learning capabilities that align with your project goals.
- Request Demos: Take advantage of free trials or demos to assess usability and functionality before making a commitment.
- Check for Support: Ensure the provider offers reliable customer support and training resources to assist your team.
- Read Reviews and Case Studies: Learn from other users’ experiences to gauge effectiveness and reliability.
Real-World Applications of Labelling Tool Machine Learning
There are numerous industries leveraging labelling tool machine learning to enhance their data annotation efforts:
Healthcare
In the healthcare industry, accurate data annotation is essential for developing diagnostic tools. Annotated medical images help algorithms learn to detect potential issues, enabling early intervention and better patient outcomes.
Automotive
Self-driving vehicles depend on annotated data to navigate effectively. Labelled images and videos create a training dataset that helps autonomous systems interpret their surroundings accurately.
Finance
In finance, annotated datasets assist in fraud detection by training models to recognize legitimate versus fraudulent transactions. Proper labelling tools can streamline this annotation process significantly.
Conclusion
The rise of the labelling tool machine learning in the data annotation domain reflects the growing necessity for precise, automated solutions that can keep up with the demands of big data. As businesses continue to embrace machine learning technologies, effective annotation will remain a critical component of their success.
Investing in a high-quality labelling tool not only optimizes data annotation processes but also enhances overall operational efficiency and effectiveness. Keeping abreast of trends and evaluating your needs ensures that you can select the right tools to support your business objectives.
For organizations looking to enhance their data annotation efforts, exploring the offerings of KeyLabs.ai, with its robust Data Annotation Tool and Data Annotation Platform, will empower you to leap forward into AI-driven success.